Parameter sniffing (for the uninitiated)
Parameter sniffing is a well-known issue within SQL Server. Imagine you have a query that can return a wildly varying number of rows. For example, a query that returns all orders in your system for a given customer. Most of your customers have a few orders, several have a lot, and one huge customer has tons of orders.
When the query is executed for the first time, SQL Server will estimate the number of rows the query will return based on the customer_id and create a plan based on that estimate.
If you wanted the details for a small account, the plan will be optimized for a small number of rows (assuming your statistics are accurate) and will be more likely to have nested loops and key lookups. Those operations are fine for small result sets but less efficient for large result sets.
If you called to get details for your largest account, you’ll get a plan that is optimized for a huge number of rows; likely using an index\table scan or maybe a hash match join instead of nested loops.
But the plan will be compiled for the first execution, and hopefully reused after that. If the plan is geared toward a small result set, it will perform poorly for the large account, and vice versa.
You can minimize the effect of parameter sniffing using WITH RECOMPILE or OPTIMIZE FOR hints, but look at an example of parameter sniffing using WideWorldImporters.
USE WideWorldImporters GO CREATE OR ALTER PROCEDURE Sales.GetOrders @CustomerID INT AS SELECT * FROM Sales.Orders so WHERE so.CustomerID = @CustomerID; GO
I added some more data to the table, all under one CustomerID, to change its statistics. CustomerID 90 has been very busy.
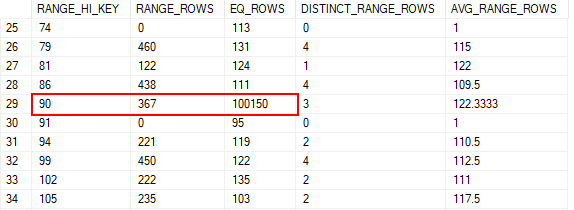
If I run this simple procedure for CustomerID 13, we’ll get a plan well suited to it, and the estimates are accurate.
EXEC Sales.GetOrders @CustomerID = 13; -- 13 or 90 GO
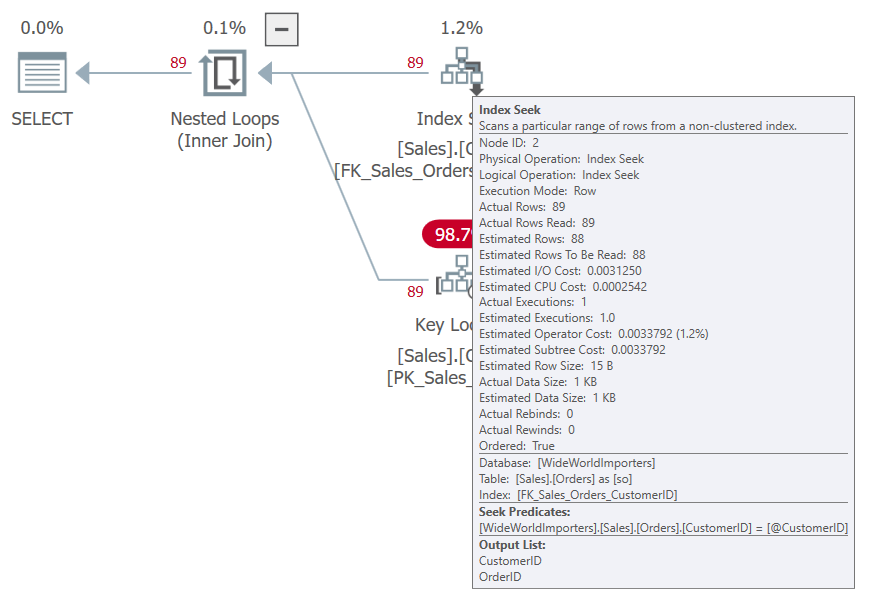
If we try again but with CustomerID 90, we use the same plan and estimate the same number of rows. But nested loops and a key lookup probably shouldn’t be the plan when we are returning over 100,000 rows.
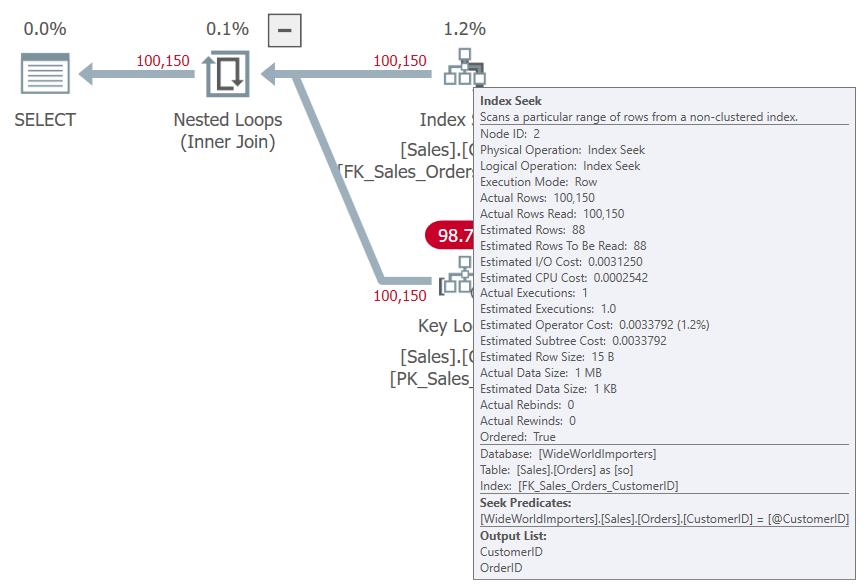
This is an example of parameter sniffing. Our plan is going to be defined largely by the parameters we use when the procedure gets compiled.
Variant Queries
Parameter Sensitive Plan Optimization attempts to solve the parameter sniffing issue by allowing a query to have different plans that are used based on the cardinality of one important parameter. The parent query has a plan that is really just a stub; there can be up to three variant queries that have full execution plans. When the query is executed, the optimizer chooses which variant query and plan to use based on the cardinality of the parameter’s value.
The plans for the parent and variant queries all indicate that PSPO is in use, and show the boundaries for the parameter. There is a low boundary and a high boundary. If the value provided for the parameter should return a small number of rows, less than the low boundary, the appropriate plan is used. If the cardinality for that value is between the two boundaries, another plan is used. If the cardinality is above the high boundary, the third plan is used.
Example
I tried several times but was unable to get PSPO to kick in on this table in WideWorldImporters, so I shifted to using the StackOverflow2013 database.
USE StackOverflow2013; GO ALTER DATABASE CURRENT SET COMPATIBILITY_LEVEL = 160; /* 2022 */ GO ALTER DATABASE StackOverflow2013 SET QUERY_STORE = ON; GO ALTER DATABASE StackOverflow2013 SET QUERY_STORE (OPERATION_MODE = READ_WRITE, QUERY_CAPTURE_MODE = ALL, INTERVAL_LENGTH_MINUTES = 15); GO EXEC DropIndexes; GO IF NOT EXISTS( SELECT 1 FROM sys.indexes si WHERE name = 'IX_User_Reputation' ) BEGIN CREATE INDEX IX_User_Reputation ON dbo.Users(Reputation); END; GO CREATE OR ALTER PROCEDURE dbo.User_GetByReputation @Reputation int AS SELECT u.AccountId, u.DisplayName, u.Views, u.CreationDate FROM dbo.Users u WHERE u.Reputation=@Reputation; GO
I’ve seen a few other blogs use Reputation as an example, and it seems its histogram is in a good state for the optimizer to choose PSPO.
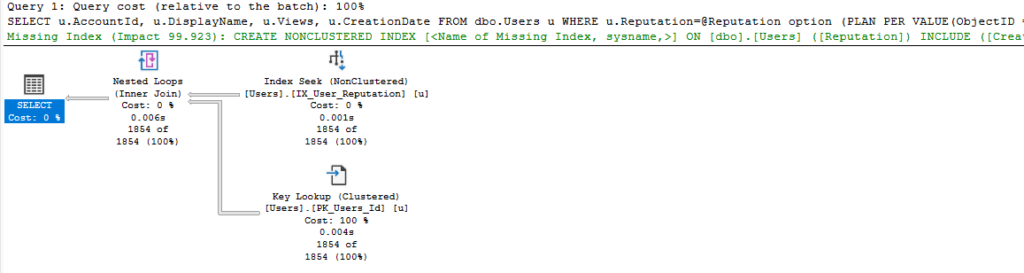
You can see from the query text at the top of the plan that the system has added an OPTION hint for “PLAN PER VALUE”. This is specifying which of the variant plans to use for this parameter.
If you look in the XML, you will also see a block like this one when PSPO is in use:
<StmtSimple StatementCompId="4" StatementEstRows="7173" StatementId="1" StatementOptmLevel="FULL" CardinalityEstimationModelVersion="160" StatementSubTreeCost="21.8786" StatementText="SELECT 
 u.AccountId,
 u.DisplayName,
 u.Views,
 u.CreationDate
 FROM dbo.Users u
 WHERE 
 u.Reputation=@Reputation option (PLAN PER VALUE(ObjectID = 1237579447, QueryVariantID = 2, predicate_range([StackOverflow2013].[dbo].[Users].[Reputation] = @Reputation, 100.0, 1000000.0)))" StatementType="SELECT" QueryHash="0x08FD84B17223204C" QueryPlanHash="0x2127C7766B9DDB3C" RetrievedFromCache="true" StatementSqlHandle="0x0900A6524A0ECC5A61EA55C6320D3963E1D20000000000000000000000000000000000000000000000000000" DatabaseContextSettingsId="1" ParentObjectId="0" StatementParameterizationType="1" SecurityPolicyApplied="false"> <StatementSetOptions ANSI_NULLS="true" ANSI_PADDING="true" ANSI_WARNINGS="true" ARITHABORT="true" CONCAT_NULL_YIELDS_NULL="true" NUMERIC_ROUNDABORT="false" QUOTED_IDENTIFIER="true" /> <Dispatcher> <ParameterSensitivePredicate LowBoundary="100" HighBoundary="1000000"> <StatisticsInfo Database="[StackOverflow2013]" Schema="[dbo]" Table="[Users]" Statistics="[IX_User_Reputation]" ModificationCount="0" SamplingPercent="100" LastUpdate="2023-05-19T14:04:14.91" /> <Predicate> <ScalarOperator ScalarString="[StackOverflow2013].[dbo].[Users].[Reputation] as [u].[Reputation]=[@Reputation]"> <Compare CompareOp="EQ"> <ScalarOperator> <Identifier> <ColumnReference Database="[StackOverflow2013]" Schema="[dbo]" Table="[Users]" Alias="[u]" Column="Reputation" /> </Identifier> </ScalarOperator> <ScalarOperator> <Identifier> <ColumnReference Column="@Reputation" /> </Identifier> </ScalarOperator> </Compare> </ScalarOperator> </Predicate> </ParameterSensitivePredicate> </Dispatcher>
The “ScalarOperator ScalarString” shows us the column and parameter our plan optimization is focusing on. We can see the index\statistic being used after the StatisticsInfo clause a few lines above; it also shows the sampling rate for that statistic.
The LowBoundary and HighBoundary values define the three ranges for parameter-sensitive plan optimization. If the optimizer estimates rows below the LowBoundary (which has always been 100 in examples I have seen), we will use the first variant. If the estimate is between the boundaries, we use the second. If it’s above the HighBoundary (which has been 100,000 or 1,000,000 in every case I’ve seen), we use the third.
In this case, we used VariantID 2 because we estimated 7173 rows.
One Problem
But as Brent Ozar points out here, there is still parameter sniffing going on. And if you are reading about the subject here, you should also read Brent’s post.
The low boundary I’ve always seen is 100, but there may be some variety there. So, if a query is executed using PSPO with a parameter that has a cardinality of less than 100, SQL Server will use the plan for that smallest range. If the parameter leads to an estimate of 5 rows, we’ll compile and execute that query and reuse the plan for subsequent low cardinality parameters. If the query is executed again with a parameter that should read 80 rows, we’ll use the same plan. But I would expect the optimal plan for those two parameters to be the same, or at least fairly similar. Nested loops and a key lookup won’t hurt here, since we aren’t talking about many rows in either case.
I would think the same for the high boundary, which I’ve seen as 100,000 or 1,000,000 in every case I’ve seen so far. Let’s assume the high boundary is 100,000. If the query is executed, and we estimate 250,000 rows to be returned, we’re likely to have a plan with a scan or hash match in it; maybe we’ll see parallelism. If the query is executed again and 1.5 million rows are expected, the plan is likely to be similar even if the memory allocation is too low.
My concern is for the middle range. A plan intended when we estimate 200 rows to be returned should be very different from a plan expecting 90,000 rows. So it seems more likely that there will be parameter sniffing in that middle range because there’s a large relative difference between the cardinality values within that range. So if parameter sniffing has a minimal effect on the high and low ranges, the improvement made by PSPO for a given query will depend on how many values fall into that middle range.
Summary
I wanted to do a post on the topic as is, before going into a problem that is potentially serious. I’ll cover that in the next post where I will talk about the changes in Query Store related to Parameter Sensitive Plan Optimization. There are changes in other system tables, but I will focus on Query Store because I use it daily.
You can follow me on Twitter (@sqljared) and contact me if you have questions. My other social media links are at the top of the page. Also, let me know if you have any suggestions for a topic for a new blog post.